Research Data Management
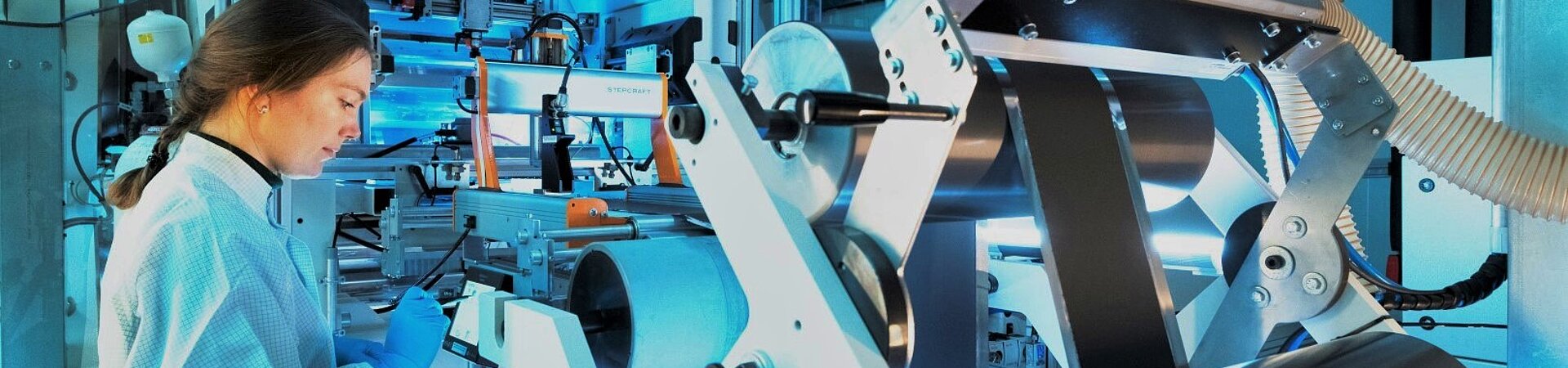
Theory and Methods
Basics
In the rapidly advancing field of battery research, from experimental breakthroughs to numerical simulations, the generation, handling, and management of research data are crucial. Whether you're a PhD student delving into your first project or a seasoned professor leading a team, grasping the fundamentals of Research Data Management (RDM) can significantly enhance the impact and efficiency of your work. This section will explore what research data entail, why managing them is essential, and how adopting the FAIR principles can benefit your research and the broader scientific community.
What Are Research Data?
Research data are the backbone of scientific inquiry. They encompass all forms of data generated or utilized in the context of research, from raw measurements and observations to processed datasets and results from simulations. In the realm of battery science, this can include:
- Experimental Data: Measurements from electrochemical tests, material characterizations, or degradation studies.
- Observational Data: Records of battery performance under various operational conditions.
- Simulated Data: Outputs from computational models predicting battery behaviors or material properties.
- Processed Data: Analyzed and interpreted data that inform conclusions and guide further research.
Beyond numerical data, methodical procedures like algorithms, software codes, and workflows are also considered research data because they are central to replicating and validating scientific findings.
The Importance of Research Data Management (RDM)
Effective RDM is the systematic approach to handling data throughout its lifecycle—from creation and collection to storage, sharing, and reuse. In the context of battery research, robust RDM practices are vital for several reasons:
- Efficiency: Well-organized data management saves time and resources by reducing redundancy and making it easier to find and use data.
- Quality and Reproducibility: Comprehensive documentation and storage practices ensure that research can be replicated and verified, enhancing the credibility of the findings.
- Data Sharing and Collaboration: Properly managed data facilitate sharing within and outside research groups, fostering collaboration and accelerating scientific progress.
- Compliance: Adherence to guidelines from funding bodies and journals regarding data transparency and archiving is essential for securing future funding and publication.
The Research Data Lifecycle
Understanding the research data lifecycle helps in planning and executing effective RDM. The stages include:
- Planning: Developing a data management plan (DMP) to outline how data will be collected, processed, and stored. This plan also addresses potential legal and ethical issues.
- Collection: Gathering data through experiments, observations, or simulations. Accurate and detailed documentation, often aided by electronic lab notebooks (ELNs), is crucial at this stage.
- Processing and Analysis: Applying methods to clean, analyze, and interpret data. Ensuring that all steps are well-documented allows others to understand and replicate the process.
- Storage: Safeguarding raw and processed data using secure and accessible storage solutions. Long-term archiving options should be considered to preserve data for future use.
- Sharing and Publication: Making data available to the scientific community via repositories and assigning persistent identifiers (PIDs) like DOIs for easy referencing.
- Reusing: Enabling other researchers to access and utilize data to generate new insights, thus contributing to the collective advancement of knowledge.
Why Adopt FAIR Principles?
The FAIR principles—Findable, Accessible, Interoperable, and Reusable—are guidelines to maximize the value of research data. Implementing these principles in battery research ensures that data are:
- Findable: Data are stored in repositories with unique PIDs and rich metadata descriptions, making them easy to locate.
- Accessible: Data can be retrieved using standardized communication protocols, and even if access is restricted, metadata remain available.
- Interoperable: Data and metadata are formatted to be usable across different platforms and by both humans and machines.
- Reusable: Data are well-documented and licensed, enabling other researchers to understand, replicate, and build upon the original work.
Practical Steps to FAIR Data Management
To make your data FAIR, consider the following steps:
- Documentation: Maintain thorough records of your data, including metadata that describe the context, quality, and structure of the data.
- Licensing: Choose appropriate licenses that clarify the terms under which your data can be reused.
- Legal and Ethical Compliance: Ensure that data sharing complies with legal and ethical standards.
- Repository Selection: Use subject-specific repositories where possible, or opt for general repositories if necessary. POLiS offers its own Kadi repository for RDM in the Cluster.
- Persistent Identifiers: Assign DOIs to your data and create an ORCID for yourself to link your contributions to your identity.
- Publication: Share your data in suitable formats and include data availability statements in your publications.
Research Software as Data
In battery research, software tools and algorithms play a crucial role in data processing and simulation. These tools are integral to scientific workflows and must also be managed according to FAIR principles to ensure that the methods are transparent and reproducible.
Support for Research Data Management
Within research organizations like POLiS, dedicated support structures are often in place to assist with RDM. These include:
- Workshops and Training: Educational programs to introduce researchers to RDM practices.
- Central Data Servers: Facilities to store and manage large datasets.
- Data Exchange Platforms: Tools to facilitate data sharing and analysis.
- Electronic Lab Notebooks: Digital tools to document and organize research activities.
- Consulting Services: Expert advice on implementing FAIR principles and complying with guidelines.
For battery scientists, effective research data management is not just a bureaucratic requirement but a foundation for high-quality, credible, and impactful research. By embracing the principles of RDM and FAIR, you can enhance the efficiency, reproducibility, and reach of your work, contributing to the global effort to advance battery technology. Investing time and resources into managing your research data effectively is an investment in the future of science.
Planning Research Data Handling
Planning and managing research data effectively from the outset of a project is pivotal for ensuring the success and integrity of your work. In the context of battery research, where data complexity and volume can be substantial, a proactive approach to research data management (RDM) is not just beneficial—it’s essential. This section delves into why planning is crucial, what constitutes a Data Management Plan (DMP), and how to create one to streamline your research processes.
Why Plan the Handling of Research Data Before Starting Research?
The importance of planning the handling of research data before embarking on a project cannot be overstated. Here’s why:
- Early Identification of Weak Points: By planning data management upfront, you can foresee potential challenges and address them before they become issues. This proactive stance helps in identifying gaps in data collection methods, storage capabilities, and security measures.
- Securing Funding: Many funding bodies now require a detailed RDM plan as part of research proposals. By including a well-thought-out data management strategy, you can request funding to cover costs associated with data storage, security, and reusability, thus ensuring financial support for these critical aspects.
- Compliance with Funding Requirements: Funding organizations often mandate clear guidelines on how research data will be managed. A well-prepared DMP aligns your project with these requirements, increasing the likelihood of receiving funding and ensuring adherence to best practices throughout the project lifecycle.
- Focus on Core Research Activities: With a clear data management plan in place, you can minimize distractions related to data security, storage, and sharing logistics. This allows you to focus more on your core research activities, enhancing productivity and innovation in your battery science projects.
What Is a Data Management Plan (DMP)?
A Data Management Plan (DMP) is a comprehensive document that outlines how data will be handled during and after a research project. It serves as a roadmap for the entire data lifecycle, detailing:
- Data Collection: Describing the types of data to be generated or used, methods of data collection, and formats in which the data will be stored.
- Data Documentation: Specifying how data will be documented, including the metadata standards to be applied, to ensure that data are understandable and reusable.
- Data Storage and Security: Outlining the strategies for secure data storage during the project, including backup procedures and data access controls.
- Data Sharing and Access: Detailing how data will be shared with the broader scientific community, including any restrictions or licenses that will govern data access and reuse.
- Data Archiving: Planning for the long-term preservation of data, specifying the repositories where data will be deposited, and ensuring compliance with any archiving requirements from funding bodies.
How to Write a Data Management Plan
Writing a DMP can be straightforward, especially with the right tools and templates. Here’s a step-by-step approach to crafting an effective DMP for your battery research project:
- Use Templates and Tools: Many funding organizations provide DMP templates tailored to their requirements. Additionally, tools like RDMO offer structured frameworks to guide you through the process of creating a DMP.
- Define Data Types and Collection Methods: Start by specifying the kinds of data you will generate or use (e.g., experimental measurements, simulation results, observational data). Describe the methodologies and technologies you will employ to collect these data.
- Plan for Documentation and Metadata: Detail how you will document your data to ensure clarity and reproducibility. Choose appropriate metadata standards relevant to your field to describe the data comprehensively.
- Outline Storage and Security Measures: Explain how and where you will store your data during the project. Include information on backup procedures and access controls to safeguard data integrity and confidentiality.
- Detail Sharing and Access Policies: Specify how you plan to share your data with the research community. This includes selecting suitable repositories, determining access levels, and outlining any licensing conditions that will apply to the data.
- Prepare for Long-Term Archiving: Identify the repositories where you will archive your data for long-term preservation. Ensure that these repositories provide persistent identifiers (e.g., DOIs) and meet the standards for findability and accessibility.
- Review and Update Regularly: A DMP is a living document. It should be reviewed and updated regularly throughout the project to reflect any changes in data management practices or project scope.
Practical Tips for Battery Scientists
- Engage Early: Begin drafting your DMP at the earliest stages of your project proposal. Early engagement with data management allows for better integration into your research workflow.
- Seek Guidance: Utilize the support services available within your institution or research cluster. Many organizations offer workshops, consultations, and online resources to help you navigate the DMP process.
- Leverage Technology: Take advantage of electronic lab notebooks (ELNs) and other digital tools that streamline data documentation and management.
- Collaborate on Best Practices: Engage with peers and data management experts to share insights and refine your DMP. Collaborative efforts often lead to more robust and effective data management strategies.
By investing time in creating a thorough Data Management Plan, you not only comply with funding requirements but also set a solid foundation for efficient, secure, and impactful research. Proper data management allows you to focus on the scientific exploration of advanced battery technologies, contributing to significant advancements in the field while ensuring that your data are preserved and accessible for future research.
Collecting Research Data
Proper data collection encompasses not only gathering data through experiments and simulations but also meticulously documenting and managing this information. This section will explore the tools and practices essential for effective data collection, with a focus on the advantages of electronic lab notebooks (ELNs) and the critical role of data documentation and metadata.
Electronic Lab Notebooks (ELNs)
Electronic Lab Notebooks (ELNs) are digital platforms that offer a modern alternative to traditional paper lab notebooks. They are designed to capture and manage the entire scientific workflow, providing a range of features that enhance data collection and collaboration. An ELN is essentially a digital version of the conventional paper lab notebook in which the entire scientific process can be captured. This digital transformation brings several significant benefits:
- Enhanced Collaboration: ELNs facilitate easy sharing and collaborative work among researchers. Unlike paper notebooks, which can be cumbersome and limited to physical access, ELNs allow multiple users to access and update information simultaneously, fostering better teamwork and communication.
- Improved Data Security and Integrity: ELNs reduce the risk of data loss associated with physical notebooks, such as misplacement or damage. Digital records are often backed up automatically, ensuring that data are preserved securely and can be retrieved when needed.
- Streamlined Reporting and Organization: Many ELNs come with built-in tools for generating reports and organizing data efficiently. These features simplify the process of summarizing and presenting research findings, saving valuable time and effort.
- Workflow Automation: Advanced ELNs, such as those integrated with platforms like Kadi4Mat, enable the creation and use of workflows—sequences of automated steps that can process data efficiently. This capability, often referred to as ELN 2.0, extends beyond simple digital note-taking to provide robust support for complex, automated research processes.
- Flexibility and Integration: While ELNs like Kadi4Mat offer sophisticated workflow management, other options such as Chemotion, elabFTW, or Jupyter provide flexibility for various research needs. These platforms can be tailored to different aspects of battery research, from chemical experiments to computational simulations.
The Role of Data Documentation
Effective data documentation is crucial for ensuring that research data are accessible, understandable, and reusable. In battery science, where data can be intricate and multifaceted, thorough documentation is essential for several reasons:
- Reproducibility: Detailed documentation allows other researchers to replicate experiments and validate findings. This is a cornerstone of scientific integrity and credibility.
- Data Discoverability: Well-documented data are easier to find and utilize. Clear descriptions of data sets, including variables and their relationships, make it simpler for researchers to locate and apply the information they need.
- Context and Interpretation: Documentation provides the context in which data were collected, which is vital for accurate interpretation. It includes information on experimental conditions, methodologies, and any assumptions or limitations that might affect the data.
Understanding Metadata
Metadata play a critical role in data documentation by providing structured descriptions of data sets. They are as important as the data themselves because they convey the essential details needed to understand, use, and reuse the data effectively. Key aspects of metadata include:
- Descriptive Information: Metadata describe the content, quality, and structure of data. This can include the type of data collected, the format in which it is stored, and the conditions under which it was generated.
- Contextual Information: Metadata provide insights into the context of data collection, such as the experimental setup, the instruments used, and the protocols followed. This information is vital for anyone attempting to replicate or build upon the research.
- Accessibility and Interoperability: Metadata enhance the accessibility and interoperability of data by detailing how data can be accessed and used. This includes specifying formats and standards that facilitate data integration across different systems and platforms.
- Enhanced Reusability: The more comprehensive the metadata, the easier it is for other researchers to understand and reuse the data. Rich metadata descriptions help ensure that data remain valuable and usable over time.
Best Practices for Data Collection in Battery Science
To maximize the quality and utility of collected data in battery research, consider the following best practices:
- Adopt and Utilize ELNs: Integrate an ELN into your research workflow to capture, manage, and share data effectively. Choose an ELN that aligns with your specific research needs and allows for collaborative work.
- Prioritize Detailed Documentation: Maintain thorough and accurate documentation of all data collection processes. Include detailed descriptions of experimental conditions, methodologies, and any anomalies or deviations encountered.
- Ensure Rich Metadata: Provide comprehensive metadata for all data sets. This should include descriptive, contextual, and technical details that facilitate data understanding and reuse.
- Leverage Workflow Automation: Where applicable, use ELNs that support workflow automation to streamline data processing and reduce manual effort. This can enhance the efficiency and consistency of data collection.
- Regularly Review and Update Data Records: Continuously update your data records and documentation to reflect the latest findings and ensure ongoing accuracy and completeness.
Collecting research data in battery science involves more than just gathering numbers; it requires meticulous planning, documentation, and the use of advanced tools like electronic lab notebooks. By adopting these practices, battery researchers can enhance the quality, reproducibility, and impact of their work, contributing to significant advancements in the field and ensuring that their data are a valuable resource for future scientific endeavors.
Analysing Research Data
Research often involves extensive data processing and visualization, using various analytical tools and techniques. To ensure the reliability and reproducibility of your findings, it is essential to document the analysis steps meticulously and leverage modern tools that support reproducible and executable workflows.
Key Steps in Data Analysis
- Data Processing: The initial phase of data analysis involves cleaning and preparing raw data for further examination. This can include:
- Data Cleaning: Removing or correcting erroneous values, handling missing data, and normalizing data formats.
- Data Transformation: Applying mathematical or statistical operations to convert raw data into a more usable form. This might involve scaling, aggregating, or filtering data sets to highlight relevant features.
- Data Integration: Combining data from multiple sources or experiments to create a comprehensive dataset for analysis.
- Data Visualization: Visualization is a powerful tool for understanding complex data and identifying patterns, trends, or anomalies. Key techniques include:
- Graphical Representations: Using plots such as line graphs, scatter plots, bar charts, and heatmaps to illustrate data relationships and distributions.
- Advanced Visualizations: Implementing more sophisticated methods like 3D plots, interactive dashboards, and network diagrams to explore data in greater depth.
- Comparative Analysis: Creating visual comparisons between different data sets or conditions to draw insights about changes or impacts.
- Statistical and Computational Analysis: Advanced analytical techniques are often necessary to extract deeper insights from data. These can include:
- Descriptive Statistics: Summarizing basic features of the data, such as mean, median, variance, and standard deviation.
- Inferential Statistics: Making predictions or inferences about a population based on a sample, using methods like hypothesis testing, regression analysis, and ANOVA.
- Computational Models: Applying machine learning algorithms, simulations, or numerical models to predict behaviors or understand underlying mechanisms in battery performance.
Documenting the Analysis Process
Accurate documentation of the analysis process is crucial for ensuring the reproducibility and credibility of research findings. Here are some best practices for documenting your data analysis:
- Detailed Workflow Description: Clearly outline each step of the analysis process, from initial data import to final visualization. Include descriptions of the operations performed, the sequence in which they were applied, and the rationale behind each step.
- Methodologies Used: Specify the statistical or computational methods employed in the analysis. This includes describing the models, algorithms, or statistical tests applied to the data.
- Tools and Software: List the software, programming languages, and tools used for data analysis. Provide version numbers and configurations to enable others to replicate your environment.
- Script and Code Documentation: For analyses conducted through programming or scripting, include detailed comments and explanations within your code. This helps other researchers understand the logic and purpose behind your code, facilitating reproducibility.
- Code Repositories: Utilize platforms like GitHub or Bitbucket to host and share your analysis scripts. These repositories can provide version control and collaboration features, ensuring that code is accessible and well-maintained.
- Data Provenance and Metadata: Maintain comprehensive metadata that describe the data sources, transformations, and analysis steps. This includes documenting the origin of the data, any preprocessing applied, and the context of the analysis.
- Provenance Tracking: Implement tools or systems that track the lineage of data from its raw form through all stages of processing and analysis. This can include automated logging of data transformations and analysis steps.
Leveraging Kadi4Mat for Reproducible Analysis
Platforms like Kadi4Mat offer robust solutions for managing and documenting the data analysis process. As part of its Electronic Lab Notebook (ELN) capabilities, Kadi4Mat provides features that enhance the reproducibility and execution of analytical workflows:
- Workflow Automation: Kadi4Mat supports the creation and execution of automated workflows that can replicate analysis processes consistently. This ensures that analytical procedures can be rerun with precision, facilitating reproducibility.
- Reusable Workflows: Define and save analysis workflows within Kadi4Mat that can be reused across different projects or experiments. This standardizes analytical practices and saves time in reimplementing common procedures.
- Integrated Documentation: The platform allows researchers to document each step of the analysis directly within the ELN. This includes embedding code snippets, output plots, and detailed descriptions of analytical methods used.
- Linked Data and Analysis: Link data sets directly to their corresponding analysis steps and results. This creates a clear, traceable connection between raw data, processed data, and the conclusions drawn.
- Executable Analysis: By supporting executable scripts and code accessing the ELN, Kadi4Mat enables researchers to run analyses directly from documented workflows. This bridges the gap between documentation and execution, ensuring that analysis steps are both reproducible and operational.
- Interactive Workflows: Use interactive workflow nodes within Kadi4Mat to perform and document analyses. These workflows can request user inputs, control devices, run code, generate plots, and display results, providing a dynamic environment for data analysis.
By meticulously documenting the analysis process and leveraging advanced tools like Kadi4Mat, researchers can ensure that their work is reproducible, reliable, and impactful. Detailed documentation, combined with the automation and integration capabilities of platforms like Kadi4Mat, empowers researchers to maintain high standards of data integrity and contributes to the advancement of battery technology.
Storing Research Data
Storing research data securely and systematically is crucial for ensuring its longevity, accessibility, and utility for future research. Data sets can be vast and complex, following best practices for data storage can safeguard against data loss and enhance collaboration and data reuse. This section outlines key considerations and strategies for effective data storage, including secure storage practices, file naming conventions, and the use of repositories and archiving solutions.
Secure Storage Practices
- Data Security: Ensuring the security of your research data involves protecting it against unauthorized access and potential hardware failures. Key strategies include:
- Access Control: Limit access to sensitive data to authorized personnel only. Use secure authentication methods and regularly update access permissions to reflect changes in your team or project.
- Encryption: Encrypt sensitive data to protect it from unauthorized access, especially when stored on external or decentralized storage media.
- Regular Backups: Implement a robust backup strategy to mitigate the risk of data loss. A widely recommended approach is to maintain at least three copies of your data:
- Primary Copy: The working copy of the data, actively used for research.
- Secondary Copy: A backup stored on a different medium, such as an external hard drive or a network-attached storage device.
- Decentralized Copy: An additional backup stored offsite or in a cloud-based storage solution. This copy ensures data safety in case of local hardware failures or disasters.
- Redundancy and Reliability: To enhance data reliability, store backups on different types of media (e.g., hard drives, SSDs, cloud storage) and use redundant storage systems like RAID configurations that provide fault tolerance.
File and Folder Naming Conventions
Consistent and meaningful naming conventions for files and folders are essential for organizing data and making it easily retrievable, both during and long after the research project. Here are some best practices:
- Use Descriptive Names: Name files and folders in a way that clearly describes their content, purpose, and context. This can include project identifiers, data type, creator’s initials, and the date or version of the data. For example:
- Project-Based Naming: BatteryProject_Experiment1_RawData_2024-07-01.csv
- Content and Creator: VoltageMeasurements_JohnDoe_July2024.xlsx
- Avoid Special Characters: To prevent issues during data processing and sharing, avoid using special characters and spaces in filenames. Use underscores (_) or camel case for separation:
- Underscore Separation: battery_test_2024_jul.docx
- Camel Case: BatteryTest2024Jul.docx
- Version Control: Implement a versioning system to keep track of different iterations of your data files. Include a version number or date in the filename to distinguish between versions:
- Version Number: AnalysisReport_v1.0.docx
- Date Stamp: ExperimentResults_2024-07-01.csv
Using Repositories for Research Data
Repositories are centralized, managed directories designed to store and describe digital objects, including research data, publications, and software. They play a crucial role in data preservation, accessibility, and sharing. Here’s how to effectively utilize repositories:
- Types of Repositories: Choose the right type of repository based on your research needs:
- Discipline-Specific Repositories: Tailored to specific scientific fields, these repositories provide specialized metadata and search capabilities that align with the domain’s standards (e.g., Dryad for biology, ICSD for crystallography).
- Interdisciplinary Repositories: These cater to multiple scientific disciplines and are useful when your data spans various fields (e.g., Zenodo, Figshare).
- Institutional Repositories: Managed by your institution or university, these repositories support institutional research efforts and often provide long-term storage solutions.
- Data Submission and Description: When depositing data in a repository, ensure it is well-documented with rich metadata. This metadata should include details about the data’s origin, methodology, and any transformations it underwent. This enhances the data’s findability and usability.
- Access and Permissions: Repositories often provide sophisticated permission management features. Decide on the level of access you wish to grant (e.g., public access, restricted access to certain groups) and configure permissions accordingly. This balances data sharing with confidentiality needs.
Long-Term Data Archiving
Long-term archiving of research data is essential to ensure compliance with DFG regulations and good scientific practices. According to these guidelines, all research data, particularly raw data, must be preserved for at least 10 years, and in some disciplines, even longer. Since it is difficult to determine the long-term relevance of data at its creation, all data should be stored without exception. If data is deleted, the deletion must be documented and justified in writing.
Key considerations for long-term data archiving include:
- Archiving Services: Utilize institutional or national archiving services that provide secure and stable storage solutions for long-term data preservation. For example, the bwDataArchive service allows KIT employees to archive their research data securely.
- Data Storage Requirements: Unlike data publication, which involves making selected datasets publicly available, data archiving involves preserving all research data privately. Ensuring a clear distinction between these processes is crucial.
- Archiving Formats: Store data in widely accepted and stable formats that remain accessible and readable. Formats such as CSV for tabular data, TIFF for images, and PDF/A for documents are recommended for archival purposes.
- Metadata for Archival Data: Comprehensive metadata is essential for ensuring archived data remains understandable and reusable. Metadata should include details about the data’s context, collection methods, and any processing it underwent.
- Archiving Management: Careful consideration should be given to how data is backed up, the physical storage medium used, and how the data is documented. Effective management practices help prevent data loss and ensure long-term accessibility.
These principles safeguard research data integrity, accessibility, and compliance with institutional and legal requirements. A robust archiving strategy not only preserves valuable research contributions but also supports the long-term advancement of scientific knowledge.
Sharing and Publishing Research Data
Sharing and publishing data is an essential practice that extends the impact of your work, enhances transparency, and fosters collaboration within the scientific community. Properly managing and disseminating research data not only builds your reputation but also accelerates scientific progress by enabling others to validate, reproduce, and build upon your findings. This section explores the benefits, methods, and considerations for effectively sharing and publishing research data.
Benefits of Publishing Research Data
Carefully handling and publishing research data in a curated form offers several significant advantages:
- Enhanced Scientific Credibility: When your underlying research data are accessible, it bolsters the reliability and trustworthiness of your findings. Transparency in data sharing allows other researchers to verify your results, strengthening the scientific validity of your work.
- Increased Visibility and Citations: Data sets can be cited similarly to research publications. Properly shared and documented data contribute to your scientific reputation and can enhance metrics such as the h-index. This increased visibility can lead to greater recognition and new opportunities for collaboration.
- Facilitating Scientific Progress: Just as you benefit from accessing the data of others, sharing your data enables fellow researchers to utilize it for their own studies, promoting cumulative advancements in battery science and beyond. Open data accelerates discovery and innovation by reducing redundancy and enabling new insights.
Where to Publish Your Research Data
Choosing the right platform to publish your research data is crucial for ensuring it reaches the intended audience and is preserved for future use. Here are some key options:
- Discipline-Specific Repositories: These repositories are tailored to specific scientific fields, making them ideal for ensuring your data is easily found and used by your peers. They often provide specialized metadata standards and search functionalities that align with the needs of your research community. To find an appropriate repository, tools like Re3data can be invaluable. Examples include:
- ICSD (Inorganic Crystal Structure Database): For crystallography and materials science data.
- The Materials Project: For a wide range of scientific data for materials.
- Interdisciplinary Repositories: When a discipline-specific repository is not available, interdisciplinary repositories are a versatile alternative. They cater to a broader range of scientific fields. Zenodo is one of the most prominent platforms for published data, offering robust support for a variety of data types and disciplines. Kadi4Mat is an indisciplinary repository for warm data (that has not been published yet) and offers a direct export to Zenodo.
- Journal-Integrated Repositories: Some scientific journals provide the option to upload data directly during the publication process. For instance, Mendeley Data supports data sharing linked to journal articles, facilitating seamless integration of data with the corresponding research paper.
- Institutional Repositories: Many universities and research institutions offer their own repositories for storing and sharing data. These repositories often provide services tailored to the needs of their affiliates. Examples include:
Licensing for Data Sharing
Choosing the right license for your data is crucial for defining how it can be reused by others. While no universal answer fits all cases, generally, the fewer restrictions you place on your data, the easier it is for others to reuse and build upon it. The widely used Creative Commons licenses provide flexible options ranging from very permissive to more restrictive terms. Tools like the Public Licence Selector can help you determine the best license for your needs.
Persistent Identifiers and ORCID
- Persistent Identifiers (PIDs): A PID is a long-lasting reference that uniquely identifies a digital object, such as a dataset or publication. The most commonly used PID in academia is the Digital Object Identifier (DOI). Assigning a DOI to your data ensures it can be consistently found, cited, and linked back to its source.
- Open Researcher and Contributor IDentifier (ORCID): An ORCID provides a unique identifier for researchers, distinguishing you from others with similar names. It is increasingly required by journals during the submission process and helps to clearly associate your data and publications with your professional identity.
Embargo Periods for Research Data
In some cases, it may be appropriate to delay the public release of your data. An embargo period is a designated timeframe during which access to the data is restricted. This allows you to finish analyzing the data or prepare additional publications before others can use it. Despite the embargo, metadata about the data can still be made publicly available and the data can be cited via its PID, ensuring visibility even during the restricted period.
Data Journals
Data journals are specialized publications that focus on the detailed description of datasets rather than conventional research findings. They provide comprehensive documentation about how data were collected, processed, and how they can be reused. Examples include:
- Scientific Data: A journal that publishes descriptions of scientifically valuable datasets.
Sharing and publishing research data thoughtfully and systematically can significantly amplify the impact of your work in battery science. By making data accessible, well-documented, and discoverable, you contribute to the collective advancement of knowledge and foster a more open and collaborative scientific environment. Whether through discipline-specific repositories, interdisciplinary platforms, or integrated journal services, the strategic dissemination of your data can enhance your research's credibility, visibility, and lasting influence.
Reusing Research Data
Reusing existing research data is a powerful strategy that can significantly expedite your own research process, allowing you to build on the foundations laid by others and potentially uncover new insights. Leveraging previously collected data saves time and resources and fosters a culture of collaboration and openness within the scientific community.
Finding Reusable Data
To locate and reuse data relevant to your field, several resources and search engines are at your disposal:
- Data Statements in Publications: The most straightforward way to access data underpinning scientific papers is through the data availability statements often included in the publications themselves. These statements typically provide direct links to where the data can be accessed, such as specific repositories or supplementary files associated with the article.
- Subject-Specific Repositories: For finding data specifically tailored to your research area, subject-specific repositories are invaluable. These repositories are designed to house data pertinent to specific scientific disciplines, ensuring that the data is highly relevant and often accompanied by rich, standardized metadata. Tools like Re3data offer comprehensive directories of such repositories, making it easier to locate the data you need within your field of interest.
- General Data Search Engines: Several robust search engines are dedicated to locating datasets across various disciplines. These platforms aggregate data from multiple sources and provide extensive search functionalities:
- B2FIND: A search engine for finding research data collections from a range of disciplines stored across European data repositories.
- BASE (Bielefeld Academic Search Engine): A powerful search engine that indexes research outputs, including datasets, from institutional repositories and other academic sources.
- Google Dataset Search: A broad-spectrum search engine that helps locate datasets from across the web, providing access to data from various sources and disciplines.
- DataCite Metadata Search: A search service that enables discovery of datasets registered with DataCite, featuring a rich array of metadata to facilitate finding the most relevant data for your research.
By utilizing these tools and repositories, you can effectively tap into the wealth of existing data, augmenting your own research with valuable resources that have already been meticulously collected and curated by others. This approach not only enhances the efficiency and scope of your work but also contributes to the overall progress of the scientific community in battery science and beyond.